Why do we need persona-based solutions to detect crime?
Human trafficking has become a $150 billion-a-year industry. There’s a new victim every four seconds. We believe that persona-based typologies are the game changer for the detection of human crimes in financial data.
Human trafficking rarely gets the attention it deserves. Did you know that there are more slaves now than there have ever been at any point in history? Human trafficking has become a $150 billion-a-year industry, with a new victim every four seconds. By the time you’ve read this intro, that’s three more people – and what about by the end of this article?
Despite the scale, and the ongoing suffering of countless individuals, the data tell a story of missed chances and ruined lives. There were just 10,572 global prosecutions reported in 2021 at a time when over 50 million people were living as slaves. And just 0.2% of human trafficking victims are ever saved.
This is a crime that happens all around us, all the time. The signs are there if you know where to look. Criminals have to find legal channels for sequestering their illegally gained money, so the key to finding the people behind the transactions lies in banking data.
We believe that persona-based typologies are the way forward. To understand what this means, it’s helpful to look at what came before. To find suspicious payments, most banks typically look at behaviour in isolation, which is known as a rules-based typology.
However, this kind of monitoring is simply too noisy. In fact, over 95% of alerts are classified as false positives in a typical rule-based financial investigation unit. And, even when a match is genuine, it is often far from clear what the actual risk is. What does it actually mean when a customer is receiving frequent low-value payments in the small hours of the morning? Are they exploiting workers – or is this something entirely different and legitimate? You can even start to wonder how many of your own transactions would fall under the purview of such a system.
And that’s why looking at behaviour is critical. Nothing we do exists in a vacuum – it’s all about context.
To give an example, someone who is aged over 35 that signs up to an online gaming service could be looking for a way to contact minors – or they may have simply just taken up a new hobby. We need to ask ourselves, when exactly does an action cross the line from legitimate to illegal? Say that same person signed up to Xbox Live, but they also bought a VPN service and regularly send money to the Philippines, a known hotbed for exploiting minors. Then it starts to look suspicious.
We call these interlinking factors red flags. RedCompass Labs has identified over 350 of these, based on years of research, validating them in conjunction with academics and through our work with a tier one bank, in which we screened a year’s worth of data from a handful of African countries.
What are financial crime personas?
Personas can be described by collections of red flags. For a given crime, multiple roles often exist: trafficker, facilitator, recruiter, and so on. And, within that, each role could have multiple personas. Each persona leaves a distinctive data footprint.
Figure 1 Mapping out the personas involved in sexual exploitation
But these personas won’t look the same in each part of the world. Banks need to map out the processes, roles, and organisations and how they work. For example, in online exploitation, this means understanding the people involved in grooming victims, as well as the technologies, payment rails, locations, and keywords that define how the business operates.
Understanding how the wider population lives is equally important, as it allows you to understand not only the environment the criminals are operating within, but how people act in everyday life. It may be quite normal to exchange large volumes of money in cash, say for wedding gifts, or prostitution may be legal.
Once the relevant personas are identified, a model can be created – this is simply a way of representing how each actor and process works together – before being tested and refined. Another seemingly incidental benefit of using persona-based typologies can be realised during this stage. In a rules-based model, false positives, which make up almost all the flagged transactions, are discarded. In this behaviour-based model, they can be turned into a strength. Patterns exist among false positives. And once a pattern is established, it can be modelled and managed.
Banks’ current methods of pinpointing suspicious transactions are falling so far short, it is essentially letting criminals get away with these heinous crimes. But we can do better.
The scale of the task seems overwhelming. It’s like looking for a needle in a haystack – with millions of haystacks appearing every second. That’s part of the reason why banks need to be proactive and stay ahead of criminals’ constantly evolving methods.
Looking at behaviour and personas just makes sense. And, powered by AI, it surmounts what seems like the insurmountable. Compared with rules-based solutions, it is like the difference between night and day. Banks can make meaning out of what seems like chaos, using data that originally would have been discarded to establish new personas – turning a former weakness into a strength. And by creating fewer but more accurate SARs, analysts’ and authorities’ time is put to better use.
This is a relatively new area of research, but multiple industry studies show that advanced behavioural analytics, when combined with entity resolution and network generation capabilities, provide the best way of targeting suspicious activities.
Share this post
Written by
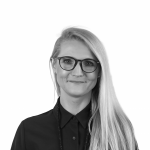
Silvija Krupena
Head of Financial Intelligence Unit
Resources